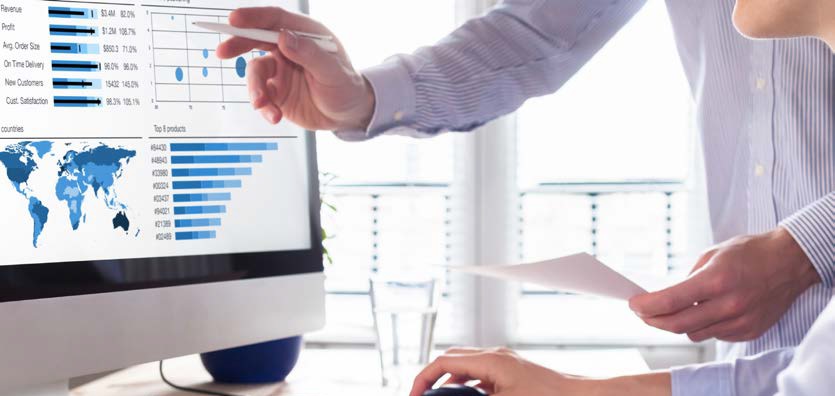
In the last few years, passive analysis of network traffic has become a challenging task due to the high variability of organisations’ IT networks. This often makes classical signature or even statistical detection approaches not sufficiently accurate in detecting potentially anomalous or malicious traffic, due to the lack of focus on network users’ behavioral analysis.
For this particular reason, the disciplines of machine learning and data mining have become increasingly appealing in solving several types of cyber security problems. In fact, passively analysing network traffic in order to identify and assess potential anomalies can be greatly assisted by employing tools obtained from the Big Data world. In this case, network traffic analyzers provide huge amounts of data per second, that can be used to train machine learning algorithms to learn what can be defined as “normal” behaviour of a network and determine what, instead, is distant from this baseline and can therefore be considered potentially malicious.
Machine Learning can be considered a powerful tool to extract meaningful information and build models of users’ behaviour but it does have some drawbacks. Data might in fact be corrupted or noisy and models’ creation may bring a high false positive rate. This limitation can be mitigated first by choosing descriptive features to be given to the algorithm, and second by integrating the contribution of different algorithms in order to make the structure more robust. Another possible solution is to create models not only of single network users but also of groups of users sharing some common behavioural characteristics.
Nonetheless, the problem of false positives is particularly true when the models’ creation is unsupervised, i.e. no data labeling is required and no additional information is provided. In this case we might not know a priori if patterns are malicious or not. Although the supervised machine learning approach is usually more effective due to the additional information, the unsupervised approach enables identification of 0-day attacks and malware not seen before, for which no information can be provided. Therefore the unsupervised approach enables the creation of algorithms that self learn the behaviour of a network, spot unusual activity, and automatically detect patterns and relationships without a priori information or human input…Click here to read full article.