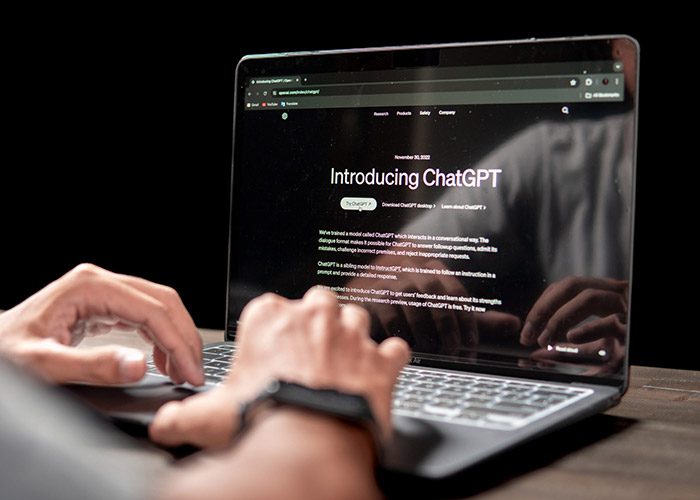
By Dr Michael Coole, Dr Warren Doudle, Nicola Lockhart, and Simeng Yuan
This article reviews the negative impact of artificial intelligence (AI) on academic integrity and learning efficacy in Australian higher education. The progression of AI technologies including ChatGPT has provided students with unprecedented tools capable of generating high-quality academic content with minimal effort or learning. This development has led to a significant increase in AI-influenced assignment artefacts, raising serious concerns about students’ mastery of essential foundational knowledge and competency in generic academic skills, along with the viability of traditional assessment methods.
The article discusses the evolution of AI use in academia, the challenges it poses to academic integrity, and the potential implications for students, educational institutions, and the professional world. This article argues that without substantial reforms in assessment practices to ensure the validation of foundational learning and development of academic skills expected at the tertiary level, the value of academic degrees will be undermined. Students who rely on AI without engaging in genuine learning may achieve academic success yet remain ill-prepared for the demands of their respective industries. This situation risks eroding trust in the competencies of graduates and questions their suitability for employment, ultimately impacting the credibility of higher education qualifications.
Introduction
Tertiary students have always faced temptations to gain unfair advantages in their academic pursuits (Bretag. 2020). The advent of the internet has facilitated the global sharing of information in seconds, often with relative anonymity (Cotton et al., 2023). The combination of the gig economy, where small quick tasks are outsourced, and the ability of enterprising students to sell their work has created an underground market within even the most prestigious universities in Australia (Lancaster, 2020).
This landscape has been further complicated by the mainstream introduction of AI, particularly with the evolution of ChatGPT-3.5 and its successors, which possess freely-accessible advanced reasoning capabilities. Recent research has shown that 43% of tertiary level student in the US use AI tools such as ChatGPT (Wang et al., 2024).
These developments have ushered in a new world for academics and students alike to navigate. It also creates a conundrum for employers that have an expectation of what fundamental knowledge and skills a graduate would poses in their fields to be able to assimilate more advanced knowledge from on-the-job education and training once hired effectively.
Early AI Use
The use of ChatGPT-3.5 and similar technologies exploded onto the university scene in late 2022, and by mid-2023, it had become a common tool in students’ assignment preparation (Lee et al., 2024; OpenAI, 2023). However, its limited reasoning abilities made it unsuitable for many assignment tasks. Nevertheless, universities struggled to implement rules governing AI use, and industry software including Turnitin developed early solutions to detect AI usage. Unfortunately, these solutions had high false-positive rates, leading many institutions to disable the feature (Turnitin, 2024).
While Turnitin has since refined its capabilities, universities remain reluctant to enable detection software, instead opting to have students reference AI use and create guidelines that are arguably subjective and easy for students to manipulate. This has created an environment where work may be heavily influenced by AI, but academic misconduct cannot be conclusively proven (Cotton et al., 2023; Ferguson et al., 2023).
The indicators of AI-generated content, such as superficial discussions of subjects and the use of specific keywords, made identifying AI use relatively easy, but not sufficient for proving academic misconduct (Kumar & Mindzak, 2024). Nonetheless, students often suffered from lower grades due to the superficial content provided by AI. Additionally, early AI models had faults, such as creating incorrect references or fabricating information when lacking a good solution, which was the easiest method of prosecuting academic misconduct (Dawson & Sutherland-Smith, 2018).
It was also common for generative AI systems to produce “hallucinations”- errors that appear plausible but are misaligned with factual information (Walczak & Cellary, 2023). However, this changed with the update of ChatGPT-4 and its advanced reasoning models.
The New Generation of Advanced Reasoning AI
As universities began to accept the new normal and work through terms such as the ethical use of AI, the next generation of advanced predictive reasoning AI was developed. Tools including ChatGPT-4, with the benefit of years of growth and development, provided students with an enhanced ability to input assignment questions along with clear directions, such as referencing and word counts, and receive an 80% solution that they could fine-tune and submit (OpenAI, 2023).
This advancement in capability led to an exponential increase in AI use, with recent research showing that 43% of tertiary level students in the US use AI tools such as ChatGPT (Wang et al., 2024). In addition, Lin et al reported an increase to 5% of students being reported for blatant AI use, where the entirety of the assignment was created without student input (Lin et al., 2024).
The use of AI in this form is a form of contract cheating, with the beneficiary being an IT company rather than another student, as was common in the past (Cotton et al., 2023). The temptation of an output or using AI “ethically” to create key points for an essay, or to brainstorm ideas has led to a drop in student engagement with online course content, with some students investing less than five hours for the semester (Bozkurt, 2023). While this method may assist in completing assignments, it creates a dangerous environment where students are not dedicating the recommended subject study period per week, resulting in only a superficial understanding of unit content upon completion at best.
This issue is compounded when undertaking more advanced units or facing exams and highly technical assignments that AI cannot solve, leading to disproportionate failure rates among students. Anecdotally, students from the 2024 cohort have admitted to not engaging with readings or attending lectures, instead relying on AI to summarize lecture slides, which misses significant amounts of context and content (Castelló-Sirvent et al., 2023).
This over-reliance on AI not only hampers the development of foundational knowledge but also poses significant risks when these students enter the workforce, particularly in industries where a deep understanding of the subject matter is critical.
Impact on Industry and Employment
The advent of AI and its over reliance results in employers and industries being confronted with graduates who may lack the essential foundational knowledge and skills expected in their respective fields. These fundamental competencies are crucial for effectively assimilating advanced knowledge and undertaking on-the-job training upon employment.
The emergence of inadequately prepared graduates entering critical sectors, such as security and intelligence, cybersecurity, psychology, and safety professions, raises serious professional concerns. The absence of foundational understanding in these areas can lead to significant negative consequences. Graduates may possess fragmented advanced knowledge acquired piecemeal, where their reliance on AI tools during education has hindered the development of a comprehensive grasp of their disciplines.
Furthermore, efforts to prepare students for an AI-driven workforce are compromised when their basic educational grounding is insufficient. Without a solid foundation, graduates are arguably ill-equipped to collaborate with or oversee AI technologies within professional settings.
Employers seeking individuals capable of critically evaluating and managing AI tools face a talent pool deficient in these essential skills. This disconnect between industry expectations and the actual capabilities of graduates poses a substantial threat to sectors that rely on well-educated professionals.
Traditionally, a single academic unit requires approximately eight hours of study per week, including reading, attending lectures, and completing assignments—amounting to over 96 hours dedicated to the subject over a semester. However, there is growing concern that students are now investing only four or five hours per week, or even across the whole semester, instead heavily relying on AI tools to generate summaries and answer technical questions for assignments. This significant reduction in study time undermines the depth of learning and mastery of the subject matter.
This situation is analogous to an individual watching a brief tutorial on parachuting, using AI to pass a theoretical exam, and then being expected to perform competently as a paratrooper without adequate practical training. The lack of foundational knowledge and practical experience in such critical circumstances is perilous.
In fields like national or critical infrastructure security, an intelligence or security professional without a solid understanding of fundamental principles, their lack of professional knowledge may compromise networks or inadvertently breach national security protocols. An intelligence analyst unfamiliar with essential methods of data collection and dissemination might fail to recognise critical threats. Similarly, a psychology graduate lacking a deep comprehension of psychological theories and practices could cause significant harm to patients.
These concerns are not merely hypothetical. Anecdotal reports indicate that new graduates are being placed into operational roles within days of joining governmental organisations, driven by high operational demands and staffing shortages. Furthermore, industry reports highlight staffing shortages and the increasing demand for skilled professionals in critical sectors (e.g., ISC² Cybersecurity Workforce Study, 2020). Organisations facing personnel shortfalls may expedite the onboarding process, potentially placing underprepared graduates into roles for which they are ill-equipped. This rapid deployment without sufficient foundational training exacerbates the risks associated with inadequately prepared professionals.
As industries demand more from graduates, where the erosion of foundational knowledge due to over-reliance on AI technologies poses a significant threat to both industry and society. The decline in essential skills and understanding undermines the competence of professionals and diminishes trust in their abilities, potentially leading to detrimental outcomes in critical sectors.
What the Future Holds
It has been suggested that the graduating class of 2023 may represent the last cohort of traditional university students who earned their degrees without the pervasive use of AI tools (García-Peñalvo et al., 2024). This trend extends to graduate students engaged in dissertation writing and research activities. The tertiary education sector must urgently address how AI is influencing student behaviours – not because it enhances their writing abilities, but because it diminishes the foundational knowledge they acquire during their studies (Eaton, 2020).
In response to the challenges posed by AI, industries employing graduates have proactively introduced authentic assessments devoid of technological assistance, such as pen-and-paper tests, to validate candidates’ knowledge prior to employment. While the use of AI in the workplace is expanding rapidly, the necessity for human employees may correspondingly decrease. Employers are likely to select only the most capable graduates who can efficiently verify AI-generated outputs and assess their accuracy and validity. Over-reliance on AI during university may render graduate’s unemployable or confine them to low-wage positions where their role is limited to prompting AI tools, with top-performing graduates overseeing their work (Cardona et al., 2023).
The potential harm caused by unprepared graduates is particularly alarming in critical sectors. For example, a cybersecurity professional lacking foundational knowledge could inadvertently introduce vulnerabilities into a network or fail to recognise security breaches, leading to significant financial losses or threats to national security. Similarly, psychologists or safety professionals without a profound understanding of their field might make decisions that harm individuals or fail to prevent accidents. This mismatch between industry needs and graduate capabilities poses a substantial threat to sectors that depend on well-educated professionals (Benhayoun & Lang, 2021; Mayer, 2024).
Although these graduates may appear to make significant strides initially, their deficiencies become evident over time. Genuine understanding necessitates humility and continuous learning, as opposed to superficial knowledge. This underscores the value of deep, foundational learning that has traditionally been the cornerstone of undergraduate education.
As Confucius stated, real knowledge is to know the extent of one’s ignorance (Fingarette, 2023). The term ignoramus, derived from Latin, meaning without knowledge, highlights the consequences of lacking true understanding.
Challenges in Addressing the Negative Impact of AI on Academic Integrity
Educational institutions are clearly struggling with the significant challenges posed by AI’s negative impact on academic integrity (Lee et al., 2024). While integrating AI ethics into the curriculum might seem like a sensible solution, in practice, it faces substantial hurdles. Universities often find it difficult to adapt quickly to technological changes, and many educators may lack the necessary expertise to teach these complex subjects effectively (Selwyn, 2019). Saylam et al (2023) highlight a growing concern: students might be able to meet assessment requirements without genuinely engaging with the material or even considering the ethics of using generative AI, which only deepens the problem instead of resolving it.
Redesigning assessments to outpace AI capabilities places an additional burden on already overworked academics with educators lacking the resources or support to develop innovative assessment methods (Harper et al., 2019; Swiecki et al., 2022). Even when new strategies are implemented, tech-savvy students often find ways to circumvent them using advanced AI tools. This ongoing struggle not only drains institutional resources but also detracts from the primary goal of education: developing subject matter understanding and critical thinking skills (Dawson & Sutherland-Smith, 2018).
Moreover, whilst universities are establishing clear policies on AI usage, enforcing them is a significant challenge. Eaton (2020) highlighted the commonly exploited loophole arguing that what is considered ethical use can be subjective. The rapid evolution of AI technologies means that policies become outdated almost as soon as they’re published and this ineffective policy environment lets AI misuse continue unchecked, further eroding academic integrity and undermining the value of degrees awarded (Newton, 2018).
Furthermore, relying on detection tools like plagiarism checkers often provides a false sense of security. While these tools are designed to catch instances of academic dishonesty, they often fail to keep up with the sophistication of AI-generated content (Foltýnek et al., 2019; Turnitin, 2023). Consequently, high false-positive rates can unfairly penalise innocent students, while those adept at using AI technologies slip through undetected. This situation not only undermines trust in the assessment system but also wastes valuable time and resources that could be better invested in educational initiatives aimed at promoting genuine learning (Sivasubramaniam et al., 2016)..
While some argue that AI can enhance learning experiences, in practice, it often promotes complacency and dependence (Zhai et al., 2024). Students may become overly reliant on AI for answers, bypassing the critical thinking process entirely (Kaledio et al., 2024). This dependence diminishes their educational experience and leaves them ill-prepared for real-world challenges where AI cannot substitute for deep understanding and problem-solving abilities. The erosion of foundational knowledge affects individual learners and has broader implications for the quality of the future workforce (Selwyn, 2024).
The proposed measures to combat the negative impact of AI on academic integrity, such as promoting ethical use, redesigning assessments, and developing policies, present significant practical challenges and are unlikely to provide effective solutions in isolation (Plata et al., 2023). The rapid advancement of AI technologies continues to outpace the efforts of educational institutions, leaving a widening gap in foundational knowledge acquisition (Heckler & Forde, 2014). Without significant and immediate action that goes beyond surface-level solutions, the erosion of academic integrity will persist (Paul 2024).
Over-reliance on AI hampers individual student development and poses a substantial threat to industries that depend on well-educated graduates (Abercrombie, 2023). The value of tertiary education is at risk of diminishing irreparably, leading to long-term consequences for society as a whole (Ivanov, 2023). As new graduates enter the workforce ill-prepared, trust in academic qualifications diminishes, and the potential for significant errors in critical fields increases, the cumulative effect of these challenges underscores the urgent need for comprehensive strategies that address the root causes of AI misuse in academia.
Finally, the approach undertaken by different universities to manage the integrity of learning may establish a university stratum for employers, where employers select graduates from institutions who are reputed to have robust assessment regimes such as formal examinations, evidencing formal learning over those institutions who do not.
Conclusion
The rapid advancement of artificial intelligence technologies, particularly tools like ChatGPT, has significantly impacted academic integrity in Australian higher education. The accessibility of these AI tools has led to an increase in AI-influenced assignments, raising serious concerns about students’ acquisition of foundational knowledge and the effectiveness of traditional assessment methods. Educational institutions face substantial challenges in combating AI misuse, including difficulties in policy enforcement, resource constraints, and the limitations of current detection mechanisms.
This widespread reliance on AI undermines the depth of learning and mastery of subject matter, as students invest less time and effort in genuine study. The erosion of foundational knowledge not only jeopardises individual student development but also poses a significant threat to industries that depend on well-educated graduates. Employers are increasingly confronted with graduates who lack essential skills and competencies, leading to diminished trust in academic qualifications and potential risks in critical sectors such as cybersecurity, intelligence, and healthcare.
Efforts to address these challenges, such as integrating AI ethics into curricula, redesigning assessments, and developing institutional policies, are fraught with obstacles. Resource limitations, technological advancements outpacing policy development, and the difficulty of enforcing guidelines contribute to the persistence of academic integrity issues. Moreover, the reluctance of institutions to share data and strategies due to competitive pressures hampers collaborative efforts that could lead to more effective solutions.
Without substantial and immediate action to reform assessment practices and reinforce the importance of foundational learning, the value of tertiary education degrees is at risk of being undermined. It is imperative for educators, institutions, and industries to collaborate in developing comprehensive strategies that address the root causes of AI misuse in academia. This includes creating robust assessment methods that validate students’ genuine understanding, promoting a culture of academic integrity, and preparing students to critically engage with AI technologies rather than passively relying on them.
The consequences of inaction are profound. The continued erosion of foundational knowledge will not only impair individual career prospects but also have lasting detrimental effects on society as a whole. Trust in higher education qualifications will diminish, and the ability of industries to function effectively with competent professionals will be compromised. As we navigate this critical juncture, the higher education sector must reaffirm its commitment to cultivating deep, meaningful learning.
By addressing the challenges posed by AI head-on, institutions can ensure that graduates are equipped with the knowledge and skills necessary to navigate the complexities of their respective fields safely and effectively, thereby safeguarding the credibility and value of higher education in the era of artificial intelligence.
References
Abercrombie, C. (2023). Ethics in Artificial Intelligence. Shanlax International Journal of English.
Benhayoun, L., & Lang, D. (2021). Does higher education properly prepare graduates for the growing artificial intelligence market? Gaps identification using text mining. Human systems management, 1-13.
Bozkurt, A. (2023). Generative artificial intelligence (AI) powered conversational educational agents: The inevitable paradigm shift. Asian Journal of Distance Education, 18(1).
Bretag, T. (2020). A Research Agenda for Academic Integrity. Edward Elgar Publishing. https://books.google.com.bd/books?id=9SXsDwAAQBAJ
Cardona, M. A., Rodriguez, R. J., & Ishmael, K. (2023). Artificial Intelligence and the Future of Teaching and Learning. . U. O. o. E. Technology. https://www.ed.gov/sites/ed/files/documents/ai-report/ai-report.pdf
Castelló-Sirvent, F., Félix, V. G., & Canós-Darós, L. (2023). AI In Higher Education: New Ethical Challenges For Students And Teachers. EDULEARN23 Proceedings,
Cotton, D. R. E., Cotton, P. A., & Shipway, J. R. (2023). Chatting and cheating: Ensuring academic integrity in the era of ChatGPT. Innovations in Education and Teaching International, 61(2), 228-239. https://doi.org/10.1080/14703297.2023.2190148
Dawson, P., & Sutherland-Smith, W. (2018). Can markers detect contract cheating? Results from a pilot study. Assessment & Evaluation in Higher Education, 43(2), 286-293. https://doi.org/10.1080/02602938.2017.1336746 Eaton, S. (2020). Academic Integrity During COVID-19: Reflections From the University of Calgary. 48, 80-85.
Ferguson, C. D., Toye, M. A., & Eaton, S. E. (2023). Contract Cheating and Student Stress: Insights from a Canadian Community College. Journal of Academic Ethics, 21(4), 685-717. https://doi.org/10.1007/s10805-023-09476-6
Fingarette, H. (2023). Confucius: the Secular as Sacred. Apocryphile Press.
Foltýnek, T., Meuschke, N., & Gipp, B. (2019). Academic Plagiarism Detection: A Systematic Literature Review. ACM Computing Surveys, 52(6), Article 112. https://doi.org/10.1145/3345317.
García-Peñalvo, F. J., Llorens-Largo, F., & Vidal, J. (2024). La nueva realidad de la educación ante los avances de la inteligencia artificial generativa. RIED-Revista Iberoamericana de Educación a Distancia, 27(1), 9-39. https://doi.org/10.5944/ried.27.1.3771.
Harper, R., Bretag, T., Ellis, C., Newton, P., Rozenberg, P., Saddiqui, S., & van Haeringen, K. (2019). Contract cheating: a survey of Australian university staff. Studies in Higher Education, 44(11), 1857-1873. https://doi.org/10.1080/03075079.2018.1462789.
Heckler, N., & Forde, D. (2014). The Role of Cultural Values in Plagiarism in Higher Education. Journal of Academic Ethics, 13, 61-75. https://doi.org/10.1007/s10805-014-9221-3.
Ivanov, S. (2023). The dark side of artificial intelligence in higher education. The Service Industries Journal, 43, 1055 – 1082.
Kaledio, P., Robert, A., & Frank, L. (2024). The Impact of Artificial Intelligence on Students’ Learning Experience. SSRN Electronic Journal.
Kumar, R., & Mindzak, M. (2024). Who wrote this? Detecting artificial intelligence–generated text from human-written text. Canadian Perspectives on Academic Integrity, 7(1).
Lancaster, T. (2020). Commercial contract cheating provision through micro-outsourcing web sites. International Journal for Educational Integrity, 16(1), 4. https://doi.org/10.1007/s40979-020-00053-3.
Lee, D., Arnold, M., Srivastava, A., Plastow, K., Strelan, P., Ploeckl, F., Lekkas, D., & Palmer, E. (2024). The impact of generative AI on higher education learning and teaching: A study of educators’ perspectives. Computers and Education: Artificial Intelligence, 6, 100221. https://doi.org/https://doi.org/10.1016/j.caeai.2024.100221.
Lin, X., Chan, R. Y., Sharma, S., & Bista, K. (2024). ChatGPT and Global Higher Education: Using Artificial Intelligence in Teaching and Learning. STAR Scholars Press Baltimore.
Mayer, C. (2024). Thriving in an AI-Dominated World: Why Higher Education Must Produce Graduates who are uniquely human and technically competent. International Journal of Emerging and Disruptive Innovation in Education: VISIONARIUM, 2(1), 2.
Newton, P. M. (2018). How Common Is Commercial Contract Cheating in Higher Education and Is It Increasing? A Systematic Review. Frontiers in Education.
OpenAI. (2023). GPT-4 Technical Report. OpenAI. https://cdn.openai.com/papers/gpt-4.pdf.
Paul , S. A. V. (2024). Strategies, Tactics, and Techniques to Mitigate Against AI in Tertiary Education: Preserving Academic Integrity and Credibility. Integrated Journal for Research in Arts and Humanities..
Plata, S., De Guzman, M. A., & Quesada, A. (2023). Emerging Research and Policy Themes on Academic Integrity in the Age of Chat GPT and Generative AI. Asian Journal of University Education.
Saylam, S., Duman, N., Yildirim, Y., & Satsevich, K. (2023). Empowering education with AI: Addressing ethical concerns. London Journal of Social Sciences.
Selwyn, N. (2019). Should robots replace teachers? AI and the Future of Education. Polity Press. https://www.wiley.com/en-gb/Should+Robots+Replace+Teachers%3F%3A+AI+and+the+Future+of+Education-p-9781509528967.
Selwyn, N. (2024). On the Limits of Artificial Intelligence (AI) in Education. Nordisk tidsskrift for pedagogikk og kritikk, 10, 3-14. https://www.semanticscholar.org/paper/45063d25b2015cf79a4d7a11545309e240f76b1b.
Sivasubramaniam, S., Kostelidou, K., & Ramachandran, S. (2016). A close encounter with ghost-writers: an initial exploration study on background, strategies and attitudes of independent essay providers. International Journal for Educational Integrity, 12(1), 1. https://doi.org/10.1007/s40979-016-0007-9.
Swiecki, Z., Khosravi, H., Chen, G., Martinez-Maldonado, R., Lodge, J. M., Milligan, S., Selwyn, N., & Gašević, D. (2022). Assessment in the age of artificial intelligence. Computers and Education: Artificial Intelligence, 3, 100075. https://doi.org/https://doi.org/10.1016/j.caeai.2022.100075.
Turnitin. (2023). AI writing: An annotated hotlist for educators | May 2023. https://www.turnitin.com/papers/ai-generated-text-an-annotated-hotlist-for-educators-may-2.
Turnitin. (2024). AI writing detection capabilities. https://guides.turnitin.com/hc/en-us/categories/22037225052173-Academic-integrity-tools.
Walczak, K., & Cellary, W. (2023). Challenges for higher education in the era of widespread access to Generative AI. Economics and Business Review, 9(2), 71-100..
Wang, Y.-M., Wei, C.-L., Lin, H.-H., Wang, S.-C., & Wang, Y.-S. (2024). What drives students’ AI learning behavior: a perspective of AI anxiety. Interactive Learning Environments, 32(6), 2584-2600. https://doi.org/10.1080/10494820.2022.2153147 .
Zhai, C., Wibowo, S., & Li, L. D. (2024). The effects of over-reliance on AI dialogue systems on students’ cognitive abilities: a systematic review. Smart Learn. Environ., 11, 28.