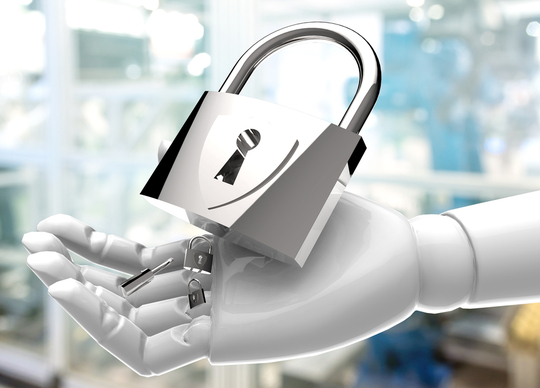
Artificial intelligence (AI), machine learning (ML), and deep learning (DL) are often applied in cybersecurity, but their applications may not always work as intended. ISACA’s new publication, AI Uses in Blue Team Security, looks at AI, ML and DL applications in cybersecurity to determine what is working, what is not, what looks encouraging for the future and what may be more hype than substance.
Leveraging interviews with some of the engineers behind these technologies, firsthand examination and use of some of the related products, and observations of chief information security officers (CISOs) and chief information officers (CIOs), AI Uses in Blue Team Security seeks to determine whether marketing tactics obscure reality when it comes to new security technology.
Of the 13 engineers who commented for this publication, none felt that the marketing associated with the products they were working on was completely accurate with respect to advertised capabilities. However, the engineers were optimistic about the direction they were heading and the technologies they would be creating as they relate to ML and DL.
The publication outlines the three areas in cybersecurity where the engineers believe that ML helps most significantly:
- Network intrusion detection/security information and event management (SIEM) solutions: Keeping an intrusion detection system (IDS) up to date can be a manual and time-consuming process. In the market today, ML capabilities are helping to enhance and reimagine the IDS methods of signature-based intrusion detection and anomaly-based intrusion detection.
- Phishing attack prevention: There are bots and automated call centres that pretend to be human; ML solutions such as natural language processing (NLP) and Completely Automated Public Turing tests to tell Computers and Humans Apart (CAPTCHAs) help prove whether users are human or a machine, in turn detecting potential phishing attacks.
- Offensive cybersecurity application: ML is being applied to help with phases of penetration testing, specifically in reconnaissance, scanning and fuzzing/exploit development.
On the other hand, there are a few areas where ML is overused. Developers may be using ML for problems that do not require it, or in some instances, ML solutions may be ineffective. The paper explores those areas as well as malicious uses of ML and DL, specifically in social engineering and phishing.
“Machine learning’s gradual adoption in cybersecurity has led to good results, and there are innovative products in the market that should take ML and DL to new levels,” says Keatron Evans, principal security researcher, Infosec, and lead developer of the publication. However, it’s possible cybercriminals may be outpacing the cyber defenders when it comes to developing and employing new technologies, and not all ML/AI-based products are as innovative as they claim to be. Cybersecurity professionals need to continuously educate themselves to be able to not only stay on top of the latest developments, but also discern which technology tools will best meet their needs.”